Obtaining patents for methods using AI to solve the client's problems
Here we introduce a senior manager who builds frameworks for making sales presentations adapted to customer attributes. The system enabled a major life insurance company to address management issues by improving policy closing rates among its sales staff.
Obtaining patents for methods using AI to solve the client's problems
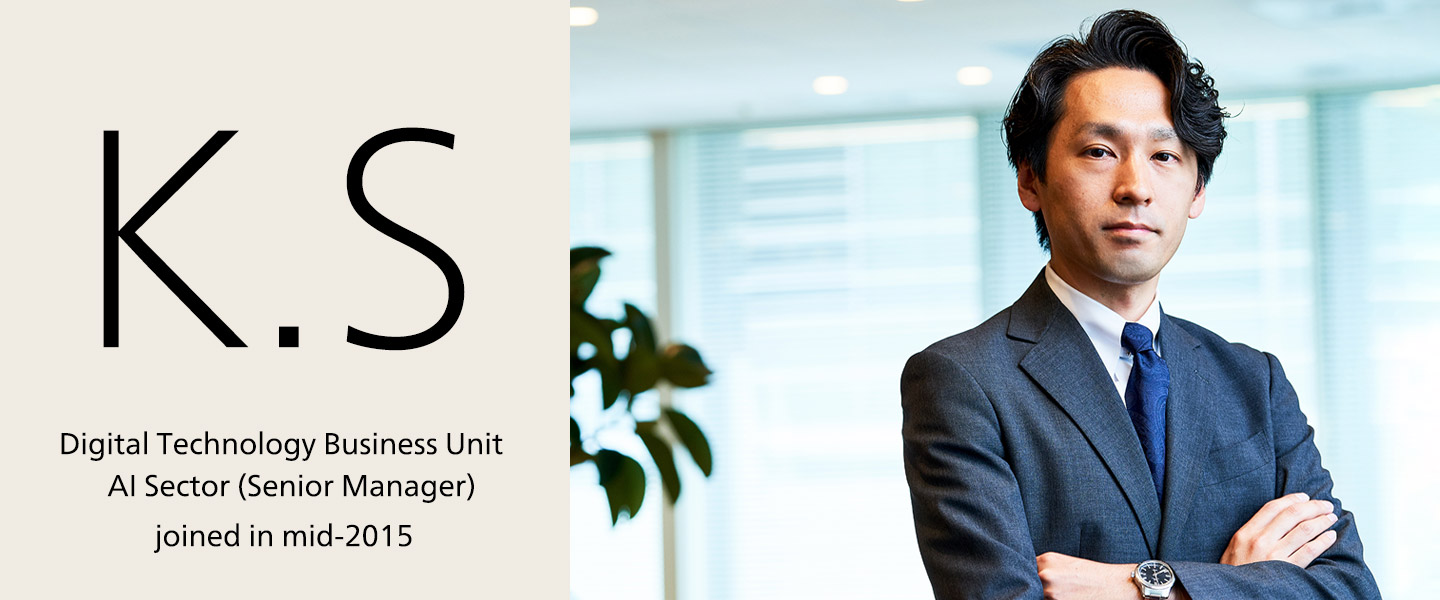
Why ABeam ?

Being a Real Partner means not stopping at recommending solutions to client management issues, but being able to support them in transformation.
My previous job was with a foreign-funded marketing research firm, where I worked in a department specializing in data analysis, mainly applying multivariate analysis and machine learning methods to analysis of measurements of promotion effectiveness and customer satisfaction survey results. I was able to learn a lot in providing clients with statistical analysis data generated using methods developed overseas.
But at the same time, it was also difficult to know how the analytical data I had worked on was being put to use. Also, the scope of impact of analytical results was limited to individual promotions. That's why I sensed the appeal of a consulting firm where I could use data to make recommendations all the way through to the solution of the client's management issue. I also decided to join because, given my experience working for the Japanese branch of an overseas firm, I figured I would be able to work with more speed and flexibility at ABeam, a global consulting firm with its head office in Japan.
Experiences in ABeam ~ Impressive Project~
The project that left the biggest impression on me was one in which we made use of AI for a major life insurance company.
One client management issue was that sales personnel increased closing rates using insurance product design descriptions adapted to the coverage needs of customers. By utilizing the enormous daily accumulation of sales presentation data, we built a framework enabling sales personnel to make maximally effective presentations tailored to their customers' attributes. Specifically, we created specifications that enabled sales personnel to automatically recommend multiple optimal patterns when producing their insurance product designs. This not only led to increased closing rates, but also to greater efficiency in the production of insurance product designs.
The biggest struggle we had in advancing this project was the extremely high level of data analysis difficulty. Since much of the data was classified according to specialized finance industry terminology, and the main sales style was that of in-person visits with customers, we had few precedents for making use of this data. So we had to start from zero in considering methods for determining what data to use and how to do the calculations to achieve higher closing rates. By then involving the client, project members, and sometimes ABeam members outside the project, we isolated from hundreds of data categories the information that contributed to product recommendations, and recommended formulae that led to optimal results.
After precise calculations and analysis, we were able to design and develop a mock up of multiple automated product recommendations expected to have high contract closing likelihoods because they were adapted to the distinctive characteristics of the customers handled by individual salespeople. And we did it in a proof of concept period of just three months. After that, it was operated in the workplace for more than a year, and has now been fully implemented in modified form. Also, the new system for recommending insurance product descriptions received a strong evaluation, and we were able to obtain a patent for it.
I think that ABeam's corporate culture was a factor in the success of this project. At ABeam, we can consult unhesitatingly with members who have not been assigned to a given project. There is a rooted culture of friendly cooperation and applying views from a variety of backgrounds. It is precisely this corporate culture that leads to solutions to the client's management issues and builds relationships of trust. With the present project as well, we made the most of ABeam's diverse range of expertise. A result of this was that we received the highest level of evaluation from the client in the post-project evaluation.
Future ~Growth Story~

Given the presence of structured data and patterned analytical themes, it has recently become possible for in-house data scientists and for citizen data scientists to handle tasks using tools like AutoML. But we also have to consider existing internal data associated with business issues, and subject it to analytical themes that take into consideration the data that will have to be collected moving forward.In doing so, people look to a consulting firm's team of data scientists to be able to envision what is to be accomplished from the analytical results, how that solution should look, and how it can be applied to business.
I would like to create teams that can address issues that are inherent in each company and industry, responding from the multiple perspectives of algorithms, strategies and IT.
At present, I am concentrating efforts to utilize voice and other unstructured data that is difficult to process. For instance, I think it will be possible to transform business itself by using natural language processing technology to extract useful information from client conversation data, and then anticipate customer needs in advance.
AI is a critical element in achieving digital transformation for the client. I hope to contribute to client transformation by leading the AI sector with a wide variety of projects involving data utilization and application.